1: Direction fields.#
Ordinary differential equations.#
An ordinary differential equation (ODE) is an equation relating the derivatives of some unknown function
The order of the ODE is highest order of derivative that appears in the equation. A typical first-order ODE can be expressed in the form
A differential equation together with one or more initial values is called an initial-value problem (IVP). Typically, the number of initial values needed to obtain a unique solution is equal to the order of the differential equation. For example, the data
define a first-order IVP with a unique solution. This course will primarily focus on the development of (approximate) numerical solutions to first-order IVPs.
Direction fields for first-order ODEs.#
A direction field (or slope field) is a graphical visualization for an ODE consisting of short line segments which are tangent to the unique solution to the ODE that passes through the midpoint of the line segment.
Given an ODE of the form (1) a direction field can be generated by evaluating
Creating direction fields with Python.#
Below we generate a direction field with window
# import numpy and matplotlib.pyplot with conventional shorthands
import numpy as np
import sympy
from matplotlib import pyplot as plt
plt.style.use("dark_background")
# define ODE RHS
f = lambda x, y: y + np.sin(x)
# set window boundaries
xmin, xmax = -3, 3
ymin, ymax = -2, 2
# set step sizes defining the horizontal/vertical distances between mesh points
hx, hy = 0.25, 0.25
# sample x- and y-intervals at appropriate step sizes; explicitly creating array of doubles
xvals = np.arange(xmin, xmax + hx, hx, dtype=np.double)
yvals = np.arange(ymin, ymax + hy, hy, dtype=np.double)
# create rectangle mesh in xy-plane; data for each variable is stored in a separate rectangle array
X, Y = np.meshgrid(xvals, yvals)
dx = np.ones(X.shape)
# create a dx=1 at each point of the 2D mesh
dy = f(X, Y)
# sample dy =(dy/dx)*dx, where dx=1 at each point of the 2D mesh
# normalize each vector <dx, dy> so that it has "unit" length
[dx, dy] = [dx, dy] / np.sqrt(dx**2 + dy**2)
# plot "vector field" without arrowheads
fig, ax = plt.subplots(layout="constrained")
# NOTE: pivot='mid' anchors the middle of the arrow to the mesh point
# the _nolegend_ flag prevents a legend object from being generated in the later merged graphic
dplot = ax.quiver(
X, Y, dx, dy, color="w", headlength=0, headwidth=1, pivot="mid", label="_nolegend_"
)
ax.set_title(r"Direction field for $y' = y+\sin(x)$")
ax.set_xlabel("$x$")
ax.set_ylabel("$y$")
plt.show()
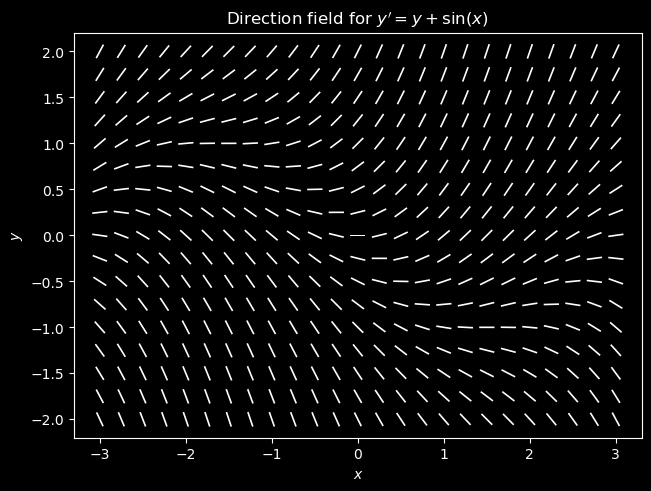
Computing symbolic solutions with SymPy.#
SymPy is a Python library for symbolic mathematics. If the ODE in question can be solved analytically, we can try using the SymPy module to compute solutions. The code snippet below demonstrates how to compute the general solution to the ODE of (2).
# redefine RHS of ODE using sympy's symbolic version of sin(x)
f = lambda x, y: y + sympy.sin(x)
# define symbolic function y and symbolic variable x
x = sympy.Symbol("x")
y = sympy.Function("y")
# create an Eq object representing the ODE
ode = sympy.Eq(y(x).diff(x), f(x, y(x)))
# solve the ODE for y(x) using sympy's dsolve
soln = sympy.dsolve(ode, y(x))
print("The general solution to the ODE")
display(ode)
print("is")
display(soln)
The general solution to the ODE
is
To solve an IVP with SymPy, we simply pass the initial conditions of the problem stored as a Python dictionary. The code snippet below computes the particular solution to the IVP (2)
x0, y0 = 0, -1 / 2
psoln = sympy.dsolve(ode, ics={y(x0): y0})
print(f"The particular solution to the IVP with initial condition y({x0}) = {y0} is")
display(psoln)
The particular solution to the IVP with initial condition y(0) = -0.5 is
We can plot the particular solution on top of the direction field that we created by first converting the solution expression to a lambda function that can be evaluated numerically.
yfunc = sympy.lambdify(x, psoln.rhs, modules=["numpy"])
xvals = np.linspace(xmin, xmax, num=100)
plt.figure(fig) # set the current figure to direction field created above
ax.plot(xvals, yfunc(xvals), color="w", label=f"${sympy.latex(psoln)}$")
ax.set_title(
f"Direction field for $y'(x)={sympy.latex(ode.rhs)}$"
"\n"
f"with particular solution when y({x0})={y0}."
)
ax.plot(0, -1 / 2, "ro") # plot initial condition point (0,-1/2) in red
ax.legend(loc="upper right")
plt.show()
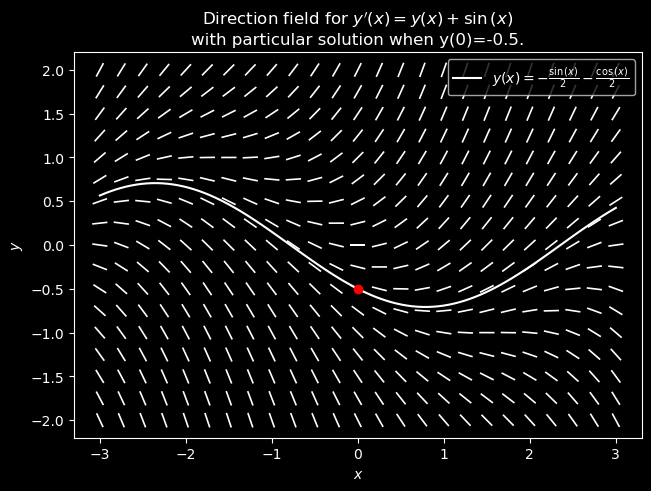